ML Algorithm Provides Insight into Immune Responses During Cancer Care
New research describes a machine-learning algorithm developed by Johns Hopkins cancer researchers that can assist in predicting patient responses to cancer immunotherapy.
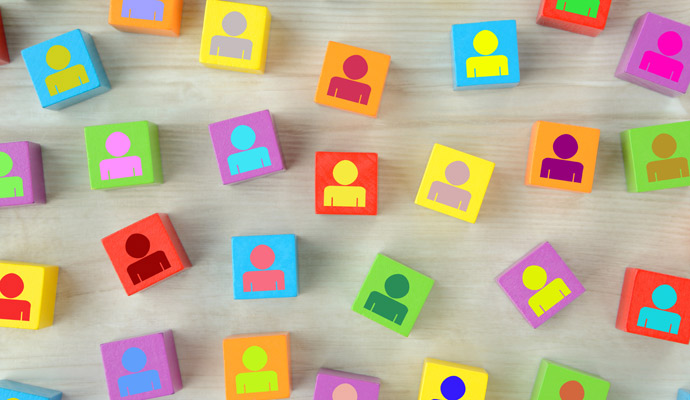
Source: Getty Images
- To help determine patient response to cancer immunotherapy, researchers at the Johns Hopkins Kimmel Comprehensive Cancer Center and its Bloomberg-Kimmel Institute for Cancer Immunotherapy trained a machine-learning algorithm to predict which patients will benefit from treatment.
In the field of cancer immunotherapy, it is often unclear which patients will experience positive results following treatment efforts, the press release notes. In this study, researchers trained a machine-learning algorithm called DeepTCR to predict how patients would respond to treatment.
Previously developed by John-William Sidhom, MD, PhD, the first author of the study, while he was a medical student at the Johns Hopkins University School of Medicine, DeepTCR can assess large volumes of data and recognize patterns using deep learning, according to the press release. Specifically, it uses data on amino acid sequences of T cell receptors (TCRs), which are located on the outside of the T cells of the immune system.
When activated by a protein from an 'enemy,' like cancer, bacteria, or viruses, TCRs will release molecules to kill the enemy. However, tumor cells can develop the ability to block the responses of T cells.
This is where various immunotherapy drugs, known as checkpoint inhibitors, can assist T cells in responding to cancer, the press release states.
In the current study, Sidhom conducted a clinical trial to determine the efficacy of one immunotherapy drug compared to a combination of two. The trial showed no significant differences between patients who were treated with one versus two drugs. Some patients responded to treatment, while others did not.
However, using genetic sequencing, Sidhom determined the type and number of TCRs in each biopsy, leading him to examine the TCR repertoire surrounding each tumor. He then fed this data to the DeepTCR program, which looked for patterns. Researchers determined that similar to known biomarkers, the DeepTCR algorithm could predict differences before treatment between the TCR repertoire in patients who eventually responded to treatment and those who did not.
"Precision immunotherapy based on the immune microenvironment in the tumor is critical to guide the optimal choice of treatment options for each patient," says Drew Pardoll, MD, PhD, professor of oncology and director of the Bloomberg-Kimmel Institute for Cancer Immunotherapy, in a press release. "These DeepTCR findings define a new dimension for predicting a tumor's response to immune checkpoint blockade by applying a novel artificial intelligence strategy to deconvolute the vast array of receptors expressed by tumor-infiltrating T cells, the key immune components responsible for direct killing of tumor cells."
Researchers also noted the potential of deep learning in improving cancer care.
"The application of the deep-learning framework in DeepTCR to characterize the TCR repertoire of T cells allows for improved stratification of patient outcomes along with model explainability in terms of identifying the predictive features," says Alexander Baras, MD, PhD, associate professor of pathology at the Johns Hopkins University School of Medicine and director of precision medicine informatics at the Johns Hopkins Kimmel Cancer Center, in the press release.
But researchers will have to confirm their findings in a larger patient population before the algorithm can be used in a clinical setting.
This is not the first time machine learning has been applied to predict patient outcomes. In June, researchers created a machine-learning model that displayed the ability to predict opioid overdose risk among Medicaid beneficiaries. After gathering data from patients with at least one opioid prescription, researchers identified various potential predictors from pharmaceutical and healthcare encounter claims. They then used this information to predict the risk of hospital visits for overdose.